Introduction. Being able to communicate in the host country language is one of the main factors of successful economic and social integration of immigrants. Language skills are a vital part of an immigrant’s human capital. Immigrants arriving in the host country before adolescence learn almost without efforts the host country language at a proficiency level almost equals that of native speakers. Although, the ability to learn new languages declines strongly with age. Immigrants arriving at an older age face greater challenges in learning the host language (Isphording, 2015; Chiswick & Miller, 2015).
Linguistic distance – the difference between two languages in vocabulary, grammar, pronunciation, and other elements of a language – strongly predicts language acquisition. The greater the linguistic distance between the immigrant’s native language and the host country language, the higher the costs associated with reaching a certain level of language proficiency (Isphording, 2015; Chiswick & Miller, 2015).
Also, engagement in language acquisition is important. Engagement signals the willingness to learn when attending host language training programmes, not merely sitting and waiting for the time to pass (Adamuti-Trache, 2013). Examples of engagement include making an effort with homework or participating in class by paying attention to the teaching (Cummins, 1981; Martinovic et al., 2009). However, the factors that influence immigrants´ engagement in host language training have been understudied.
Despite reports of drop outs, immigrants have adapted to the challenges and have come to compete as “underdogs” (Nilsson & Nyström, 2016). They have learned that they must adapt if they are to survive and must work harder and seek more help from others than natives do. Here, adult immigrants have strengths that can be used to help them; they bring with them a wealth of knowledge and experience learned through survival and transition from one life to another. Many have endured complex immigration systems and have found their way to new destinations, relying on knowledge and strategies that are not dependent on the ability to read and write (Wrigley, 2013).
Compared with natives, immigrants have a higher work devotion and tend to be highly optimistic about education. The optimism may result from migrants being essentially different from and more aspirational and driven in terms of social mobility than non-migrants (Cebolla-Boado & Soysal, 2018).
The Swedish context and language training programmes. With start in 2013 untold numbers of immigrants, mostly from Syria, began seeking asylum in Europe. Many of them arrived to Sweden. The immigrants were a quite diverse group consisting of individuals with a range of educational levels, ages, family situations, and social classes. Although many were young, without families and highly educated, others were illiterate, old, and brought family members (Nilsson & Nyström, 2016). What the immigrants had in common was that they had to learn Swedish. Education is one of the strongest pillars of the Swedish integration system. When the immigrants have received a residence permit, they are able to participate in SFI (Swedish for immigrants) as language proficiency is seen as a key to access other areas of society such as the labour market. However, there have been reports of high drop outs and SFI has been questioned by political populists (Öbrink Hobzová, 2021).
In Sweden, immigrants (18-67 years of age) who have newly arrived are offered SFI. The programme is state funded, but Swedish municipalities are responsible for providing it. The programme ensures that adult Swedish immigrants have the right to free basic language tuition up to a level corresponding to B1, as described in the Common European Framework of Reference (Council of Europe, 2001). SFI is a labour market instrument. The focus in the tuition is on work-related communication skills and periods of practical work experience (Sandwall, 2013).
Learner engagement is important because engagement contributes to learning. But how engaged are the immigrants in language training programmes? Much research has focused on student engagement in compulsory school as the key to addressing problems of low achievement, student boredom and alienation, and high dropout rates (Fredericks et al., 2004; Martin & Dowson, 2009; Naidoo, 2009; Paradis et al., 2020). Fewer studies have focused on adults’ engagement, although education in adulthood can provide greater motivation, positivity, and self-confidence for, for example, people with low education (Manninen & Meriläinen, 2011). Little to nothing is known about what factors influence the engagement of immigrants who have newly arrived when they participate in language training programmes. To my knowledge, no study has investigated the engagement of immigrants who have newly arrived in these host language training programmes and factors that influence engagement.
Purpose. The overall aim of this study was to identify predictors of adult immigrants’ engagement in host language training programmes.
More specifically, the research questions that guided the study were the following:
To what extent do socioeconomic and sociodemographic factors (sex, socioeconomic status) predict engagement in the Swedish language training programme (SFI)?
To what extent does access to human capital (HC; age, years of education) and social capital (SC; friends, neighbours, associations) predict engagement in SFI?
To what extent does academic self-efficacy for SFI predict engagement in SFI?
I have structured my article as follows: First, I discuss my theoretical framework and anchor the concepts within the current research. Second, I discuss the survey data and the methods for measuring the concepts. Third, I present the results. Fourth, I conclude with a discussion on plausible explanations for the findings and discuss how the findings can be positioned within the current research on adult immigrants’ engagement in language training programmes.
Literature review and theoretical concepts. Engagement is a multidimensional concept that includes behavioural, emotional, and cognitive components (Fredericks et al., 2004). The behavioural dimension is mostly defined in three ways: (a) positive conduct and rule following (class norms), including school attendance; (b) involvement in learning and effort, including spending time on tasks, asking questions, and contributing to class discussions; and (c) wider participation in school-related activities such as school governance (Fredericks et al., 2004). As Fredericks et al. (2004) noted, the definitions do not make distinctions among various types of behaviour, such as participation in academic and non-academic school activities.
The cognitive (psychological) dimension of engagement views engagement as an internal psychosocial process that evolves over time and varies in intensity. Research on cognitive engagement has stressed investment in learning. Although some researchers have highlighted self-regulation, strategic learning, flexibility in problem-solving, preference for hard work, effort, and positive coping with failing (Connell & Wellborn, 1991), others have stressed inner psychological quality and investment in learning (Wehlage et al., 1989). Fredericks et al. (2004) argued that effort is problematic because it is included in definitions of both cognitive and behavioural engagement.
The emotional dimension refers to students’ affective reactions in the classroom, including interest, boredom, joy, sadness, and anxiety (Fredericks et al., 2004).
There is substantial variation in how engagement has been measured. Most studies have concerned adult education as such or immigrant research (e.g., Paradis et al., 2020). Regrettably, adult education and immigration research have seldom conversed with one another. Nevertheless, I stress the importance of connecting immigrant research with research on adult education.
Several explanations of adult immigrant student engagement exist. Thus, I consider a multiple-variable framework. I begin by describing some key variables for engagement: socioeconomic and sociodemographic predictors (education, age on arrival, sex, and socioeconomic status). Thereafter, I continue with social psychological predictors.
Sociodemographic predictors and forms of capital. Consequently, the first contribution of this study is to identify predictors that can help to explain adult immigrants’ engagement in host language training (SFI). The second contribution is to advance the state of knowledge on immigrants’ engagement in Swedish language training by modelling how demographic and forms of capital variables (e.g., sex, education, age at arrival) impact attitude towards Swedish language training for immigrants.
The first explanation comes from adult education research, which has insisted on the importance of socioeconomic and sociodemographic variables (Adamuti-Trache & Sweet, 2010; Adamuti-Trache et al., 2018). Socioeconomic variables are indicated by social class or employment. Sociodemographic variables refer to length of stay, family status, education, and children. Both types of variables influence how individuals position managing to engage in learning given time (children, family) and financial constraints (social class). Social class constrains an individual’s finances, and holding a specific class position should in theory make one more risk averse as costs of educational engagement increase. Children and family imply a responsibility. A parent or spouse has to devote time to care for others (Chui, 2011)
H1: Compared with lower socioeconomic position, higher socioeconomic position corresponds with greater engagement in language training programmes.
Age and education indicate a person’s HC (Goldin, 2016). HC refers to investment in education and experience. Adamuti-Trache (2013) noted the pressure on immigrants and the host society to find ways to improve the language fluency of immigrants who have newly arrived – highly educated as well as lower educated − by engaging them in language training programmes. Highly educated immigrants are most affected by lack of language skills because many of them are qualified in occupations that require language skills in the host language (Adamuti-Trache, 2013). Typically, HC can be measured by years of schooling (investment) and age (approximate experience). An immigrant student with greater HC should have more to gain from educational engagement.
H2: Greater HC corresponds with greater engagement in language training programmes.
Next to HC comes SC, which refers to the collection of contacts (e.g., friends, peers, acquaintances). Individuals with more SC have greater access to help and expertise. For immigrant students, SC can mean help with coursework, finding a job, and opportunities to practice the native language.
H3: Greater social capital corresponds with greater engagement in language training programmes.
Access to SC and HC has been prominent in the immigration research literature. Research has almost become dominated by these two predictors (Adamuti-Trache, 2013; Adamuti-Trache et al., 2018; Boeren et al., 2010).
Social psychological predictors: Academic self-efficacy. Beyond sociodemographic variables and socioeconomics, one must consider the social psychology of the students. Social psychological explanations emphasize either humanistic or socio-cognitive theories. The former stress motivations such as needs or mindsets and the latter stress individuals’ self-efficacy − that is, the belief in one’s capacity for active tasks (Bandura, 1986). I argue for the importance of the latter theories.
Self-efficacy offers an optimistic perspective on people (Bandura, 1986). Whereas sociodemographic and socioeconomic predictors capture constraints on the individual, self-efficacy indicates people’s beliefs about their capacity to accomplish tasks given the constraints of society. Here, the concept of self-efficacy measures aspects of what Giddens (1984) referred to as “agency” (i.e., individuals’ beliefs in their capacity to act). Thus, self-efficacy may explain why some people overcome societal constraints. The statement “Believe in yourself” exercises a powerful force that cannot be overlooked as a gimmick, and self-efficacy may be useful to explain both attitudes and behaviour.
H4: Greater self-efficacy corresponds with greater engagement in language training programmes.
Self-efficacy may be general or domain specific. The key domain for immigrants in language training would be their academic self-efficacy for SFI. Those with greater self-efficacy for language training should have a higher level of engagement.
Self-efficacy grows out of social processes. Individuals gain self-efficacy from experience, such as interacting with or watching other people (Bandura, 1986). Thus, self-efficacy offers an explanation as to how cognitive and social processes relate to one another. Self-efficacy should predict an individual’s engagement in language training but not necessarily their mastery of a language. Obviously, a person can be overconfident but not overly engaged in language training.
Method. In this section, I present how the participants were sampled. Thereafter, I discuss the variables used in the study, which were derived from a survey. Finally, I discuss the strategies used for the data analysis.
Participants. The participants (n = 186) − many of them refugees − were recruited purposefully for the study from one learning centre and thus were non-random. The purpose was to sample a hard-to-reach population, meaning that the participants in the study would typically be non-respondents in general household surveys. Therefore, one could not have administered a random-sample survey to the study population and have expected a high and unbiased response rate. To clarify, some of the participants had never seen a survey before. Other participants were illiterate or lacked the language skills to comprehend the survey. Accordingly, most Swedish studies of the immigrant population tend to be qualitative because of the difficulties in reaching the population with household surveys (e.g., Sandwall, 2013; Wedin & Norlund Shaswar, 2019).
The vast majority of the participants were refugees from Syria, Somaliland, Iran, or Iraq. However, I do not have an estimate of the specific ethnic origins of the participants. The illiteracy of several of the participants posed a methodological challenge. Although the survey could have been translated into the native tongue of the participants, I would still have faced a problem if participants were illiterate and thus could not read the items. Another issue is whether the participants had the same cultural comprehension of the survey items. For example, if a person has never completed a survey, they might experience difficulties comprehending the meaning of sematic differentials (e.g., adequate/inadequate on my 1-7 rating scales). Accordingly, the issues of comprehension problems had the potential to contribute to systematic response biases in the coefficients and intercepts. My solution was to administer a survey at the learning centre and to let a teacher who was native speaker – Somali and Arabic – verbally translate the survey for the participants and facilitate interpretation of all survey items on site. I believe that having said the native speaking teacher might have helped strengthen the trust between the participants and the researcher. Consequently, several of the participants would probably never have completed a survey administered to their household.
Variables. I begin with describing the outcome variables and then continue with the predictor variables – sex, age on arrival to Sweden, length of stay in Sweden, and years of education. Table 1 reports the means, standard deviations, and minimum and maximum.
Outcome variable. The outcome variable compromises three questions concerning engagement.
To develop a measure of student engagement, I conducted a factor analysis to validate the measure of my concept. The principal factor consisted of three variables (items) asking the participants to what extent they were engaged in their studies: (a) “I think I participate actively during the lessons (raising my hand, asking questions)”; (b) “I think I work diligently with my homework and tasks connected to school work”; and (c) “I think I really make an effort to become much better.”
All three questions focus on the behavioural qualities in the classroom: participation, effort, and diligence. The participants were able to respond on a semantic differential scale ranging from 1 to 7. The differentials were always and never.
Immigrants reported very high engagement. As shown in Table 1, immigrants averaged around 6 out of 7 on all three engagement questions.
Predictor variables. I measured academic self-efficacy for SFI with the following statements: “I can solve the task,” “I consider the tasks easy,” and “I can solve the tasks with the help of the teacher.” The participants had to respond in each case by saying how important they considered the statements on a 1–7 scale. I validated the measure with principal axis factoring (PAF). To measure education, I used the participants’ reported years of schooling, which have been used as a standard indicator of immigrant HC whereby each additional year should count as an incremental investment.
Based on the participants’ reported occupation in their home country, I classified the participants by the European Socioeconomic Classification (ESESC) schema, which is a categorical social class schema based on the concept of employment relations. The schema takes into account both (a) the specific skills needed for the job and (b) the degree of autonomy of the employee. Because my sample was small, I used a three-class schema. The first class is “salariat status.” Examples of the salariat in the sample include lawyers, teachers, social workers, and civil and professional engineers. Common to these occupations are high autonomy, high skills, and non-routine jobs. The second class is “intermediate status.” Examples of intermediate people in the sample include clerks, self-employed hairdressers, barbers, bakers, and farmers. Common to these occupations is high autonomy. The third class is “working-class status.” Examples of the working class in the sample include miners, truck drivers, janitors, and construction workers. Common to these occupations are low autonomy, low skills, and routine jobs (Rose & Harrison, 2007).
The coding of the ESESC is not perfect, as it lacks detailed information about the number of employees and managerial duties. Nevertheless, imputing the ESESC on occupations is common in empirical research. The ESESC mimics Rose and Harrison’s (2007) class schema and aims at capturing aspects such as the skill requirements, employment contracts, and difficulty in monitoring.
SC was measured using several rating scale questions. All concerned the chance to speak Swedish with friends, neighbours, children’s parents, and children’s teachers, as well as at associations. Again, I validated the measure with PAF.
Length of stay indicates the number of years in the host country. The variable measures the exposure to the host country and has often been cited as a determinant of assimilation.
To adjust for family status and parental status, I added one dummy variable (1 = yes,
0 = no) for each. Family characteristics have often been cited as a key determinant of adult learning outcomes. Sex of the respondent was measured with a dummy variable for male
(1 = yes, 0 = no).
Measurement and data analysis. In the first step, I conducted an exploratory factor analysis with PAF. Table 2 displays the factor loadings. PAF has several advantages, including (a) better performance with data of small samples compared to that of maximum likelihood and principal component methods and (b) a stronger theoretical basis (i.e., treating the principal factor as a latent variable) compared with that of principal component methods. Finally, I allowed the factors to correlate. I conducted the analysis with the psych package (Revelle, 2018) in R (R Core Team, 2018).
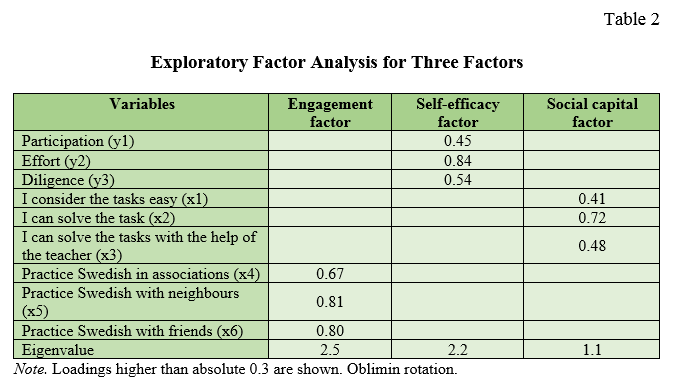
After the estimation, I computed a factor score that I used for the analysis separately for each factor. To make the factor score easy to interpret, I normalized the engagement score to range between 0 and 100.
In the second step, I selected linear regression for simplicity of interpretation. However, the outcome was skewed, which gives reason for suspicion. When plotting the residual, I found evidence of non-normality and unequal variance. This means that the standard errors would be unreliable.
To address the issue, I bootstrapped the standard errors. Bootstrapping means sampling with a replacement, which signifies that the bootstrapped sample functions as a non-parametric distribution. The bootstrapped sample is non-parametric in the sense that it relaxes distributional assumptions.
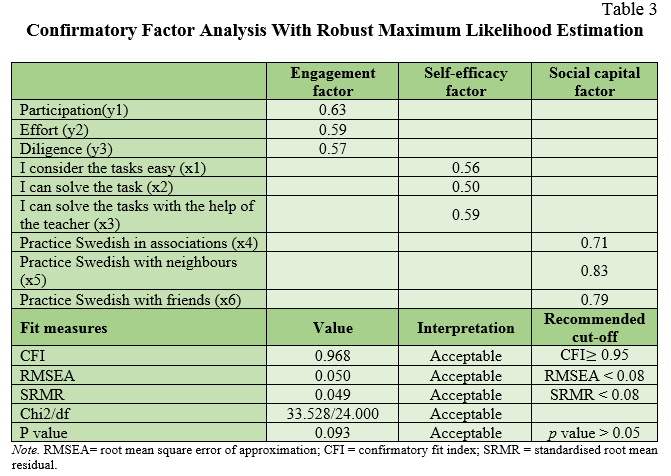
In the third step, I re-estimated the model with a confirmatory factor analysis to validate the findings (Table 3). The confirmatory factor analysis fitted well with the data. To account for non-normality, I used robust maximum likelihood estimation (West et al., 2012).
In the final step, I fitted a structural equation model (SEM). A common strategy entails adding correlations between the residuals for the item variables based on the so-called modification indices (change in chi-square statistics). However, the remedy did not work. Instead, the SEM fitted well once I removed insignificant predictors (based on the regression analysis). I conducted the analysis with the Laavan package (Rosseel, 2012) and Semplot package (Epskamp, 2015) in R.
Results. Here I will present the support for the four hypotheses in the study. Table 4 reports the linear regression models with engagement as outcome. The confidence intervals were bootstrapped. Statistically significant estimates of coefficients are indicated.
In Model 1, years of education seems marginally from zero. However, the direction of the coefficient is negative, suggesting that one additional year of education lowers the engagement by roughly 1 point, on average, after adjustments. This means that immigrants with lower education have higher levels of engagement, and vice versa. This is in sharp contrast to the story typically reported of low-skilled immigrants (cf. McQuaid et al., 2012). Considering the scale in years, 1 point is a moderate predicted difference, but far from huge.
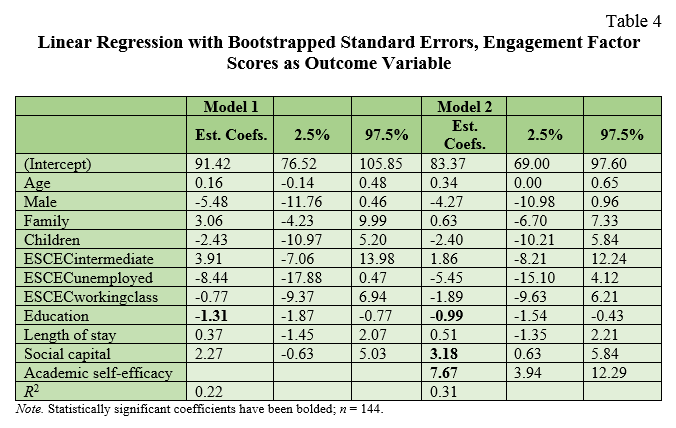
There was no statistically significant difference in engagement according to socioeconomic position. However, one must recall that I did not measure the host socioeconomic position, suggesting that the results lack support for H1.
Family, parental, or gender status does not matter in terms of statistical significance. The estimates for length of stay and age are not statistically significant. Lack of statistical significance simply indicates lack of evidence against the null hypothesis (assuming it is true). In total, Model 1 accounts for 22% of the variance in engagement (R2 = 0.22). One can interpret this as a small but noteworthy amount.
In Model 2, I added the score for academic self-efficacy. As expected, academic self-efficacy positively predicted engagement. The association was statistically significant. Students with one factor scored above the mean on academic self-efficacy and scored roughly 8 points higher on engagement, on average, after adjustments. One point in the factor score of self-efficacy can be interpreted similarly to a z score (ranging empirically from -2.4 to 0.8). Thus, the result supports H4.
However, after I adjusted for self-efficacy, the association between SC and engagement became statistically significant. This could be due to spurious correlation or data with high variability. One additional point in academic self-efficacy varied with roughly 3 points in engagement, on average, after adjustments, where one point in SC can be interpreted similarly to a z score (ranging empirically from -1.2 to 1.5). This means that the result supports H3. In total, Model 2 accounted for 31% of the variance in engagement (R2 = 0.31), i.e., moderate. Finally, note that education remained fairly unchanged. Thus, the result supports the importance of H2, but not the sign. The result goes in the opposite direction.
In the final step I fitted a structural equation model (SEM). A SEM model goes beyond exploratory analysis towards confirmatory analysis. This means that the analysis rests on theory rather than data reduction (pattern finding). The model fitted poorly when I included all predictors. However, after I used the significant predictors from the regression model, the model fit improved towards acceptable fits. I report the fitness measures in Figure 1, along with the path diagram. The edges indicate a standardized regression or loading coefficient. The squares (boxes) indicate observable variables; abbreviated with “x” and observable outcome items with “y”. Years of schooling is indicated with “Sch”. Eclipses (circles) indicate indirectly observable variables (i.e., latent variables). Latent variables are labelled “Eng” for engagement, “SE” for academic self-efficacy, and “SC” for social capital.
As can be seen in the model in Figure 1, all paths to engagement are statistically significant. One standard deviation above the mean in schooling corresponds to 0.40 standard deviations below the mean in engagement, on average, adjusting for other predictors. The magnitude of the association seems intermediate. Again, HC proves to be important. However, the surprising direction of the association leaves much to wonder about, which I return to in the discussion. The result may well be a case of regression towards the mean. Again, the result supports the importance of H2, but not the sign. Thus, the result goes in the opposite direction.
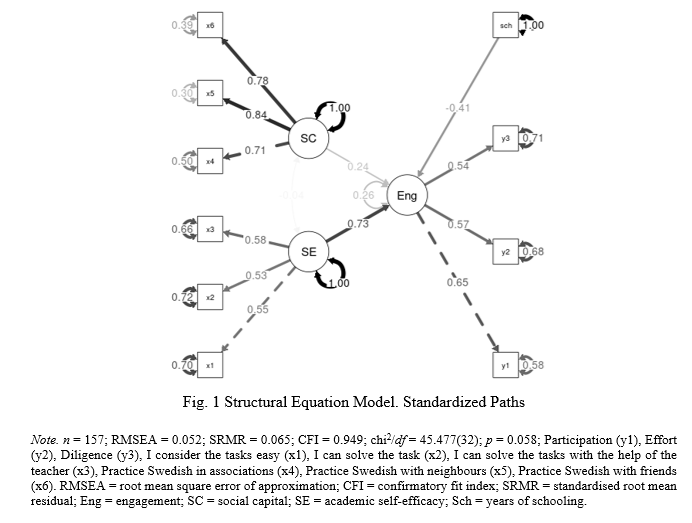
One standard deviation above the mean in SC varies with 0.24 standard deviations above the mean in engagement, on average, after adjusting for other predictors, i.e., low-to- moderate. Consequently, practicing Swedish with friends, neighbours, or members of associations promotes one’s engagement in school. The result therefore stresses the importance of SC. The result supports H3.
Finally, one standard deviation above the mean in academic self-efficacy corresponds to 0.73 standard deviations above the mean in engagement, on average, after adjustments. The magnitude of academic self-efficacy should be interpreted as huge. Thus, a word of caution seems appropriate. Large coefficients may be due to overfitting. Overfitting happens often in SEM due to the practice of adapting the model to the data. Although the fitness statistics look acceptable, one risks fitting the model too close to the data (e.g., peculiarities). Consequently, the model loses generality. Nevertheless, one should be confident in the association but careful in interpretation. Consistent with theory, self-efficacy proves to boost engagement in educational activities. The result supports H4.
Discussion. The overall aim of this study was to identify predictors of adult immigrants’ engagement in host language training programmes. The research questions guiding the study were: To what extent do socioeconomic and sociodemographic factors (sex, socioeconomic status) predict engagement in the Swedish language training programme (SFI)? To what extent does access to human capital (HC; age, years of education) and social capital (SC; friends, neighbours, associations) predict engagement in SFI? To what extent does academic self-efficacy for SFI predict engagement in SFI?
My major conclusions are as follows:
Socioeconomic position made no statistically significant difference in student engagement. Likewise, family and children made no statistically significant difference. The result indicates no support for H1.
Forms of capital made a difference in student engagement. Students with more years of education had lower engagement, opposite to H2 and HC theory. On the other hand, students with greater SC had greater engagement. The result clearly supports H3 and SC theory.
Academic self-efficacy made the largest positive difference in student engagement, consistent with H4 and socio-cognitive theory.
Although the study lacks in generality, because I included a non-random sample, the patterns point to interesting implications for adult education and immigration research. Contrary to political populist commentators, the participants reported a high level of engagement in language training (Nilsson & Nyström, 2016; Öbrink Hobzová, 2021).
In contrast to previous research on immigration and adult education, I found no relationship between socioeconomic position and engagement. Again, the results might reflect that the differences may be too small to detect in data with high variability. However, newly arrived immigrants might not resemble the population typically studied in adult education. Instead, the results support the other central concern of adult education, namely the participants’ social psychology (Adamuti-Trache & Sweet, 2010; Boeren et al., 2010).
In agreement with most of the research on immigrants, SC makes a huge difference, confirming the common belief that everybody needs a little help (Adamuti-Trache, 2013; Adamuti-Trache et al., 2018; Boeren et al., 2010). Immigrants with limited education might still commit to engagement in the presence of support (Adamuti-Trache et al., 2018; Isphording, 2015).
Opposite to the claims in immigration and adult education research, I found the opposite relationship between HC and student engagement (Adamuti-Trache & Sweet, 2010). Again, the result might be due to high variability in the data. Alternatively, the result could indicate that highly educated immigrants feel alienated in the classroom (Fredericks et al., 2004). Being forced to re-educate oneself late in life could feel demeaning. The highly educated might be distressed when they realize how long it will take before they acquire language skills needed in the host language to (a) obtain occupations they are qualified for or (b) study at a university. Some highly educated individuals probably find SFI to be a waste of time. Thus, highly educated people do not engage that much in the SFI lessons or do their homework (Adamuti-Trache, 2013).
Low-educated individuals might see SFI as an opportunity to make themselves more employable. Compared with the highly educated, they are highly optimistic about education. Swedish language skills can offer low-educated individuals a better chance at the job market and thus enable them to advance in the host society (Adamuti-Trache & Sweet, 2010; Adamuti-Trache et al., 2018; Isphording, 2015; Manninen & Meriläinen, 2011). I speculate that low-educated individuals consider education a privilege because they have not had the possibility to study in the home country. When low-educated individuals are offered the opportunity to study without costs and even receive payment when studying, they want to make the most of it (cf. McQuaid et al., 2012). Thus, low-educated individuals strive to engage in SFI and participate actively during the lessons by raising their hands, asking questions, working diligently on their homework, and making an effort to improve significantly.
As predicted by socio-cognitive theory, greater self-efficacy enables people to overcome educational constraints (Bandura, 1986). I welcome the finding as providing clear rationale for educators. Unlike socioeconomic position and forms of capital, self-efficacy consists of forces within the control of educators. Educators can promote self-efficacy in conversations by providing role models and through persuasion (Bandura, 1986). Nevertheless, I suggest that one should not overestimate the importance of self-efficacy. Clearly, the importance of forms of capital suggests that forces exist beyond the educators’ control (Adamuti-Trache & Sweet, 2010). This means that the results have bearings on conventional wisdom in adult education and immigration research.
My study is, of course, not without limitations. First, the small sample size compromised the capacity to detect small differences. Small samples may at best identify large differences (assuming they exist). Second, I did not adjust for all relevant variables. One example would be institutional variables that have been prominent in adult education research (e.g., educational tracks such as ability grouping). Another important type of unmeasured variable would be the humanistic needs of the participants. Third, all cross-sectional studies assume stability over time. Engagement might not be constant over time. Finally, I only measured one dimension of engagement. Knowledge about emotional and cognitive engagement is still lacking.
In future studies, it would be of interest to investigate newly arrived immigrants’ emotional and cognitive engagement. Another topic for future research would be to investigate if engaged low-educated individuals perform better on tests than non-engaged highly educated individuals. Furthermore, in a longitudinal study, researchers could investigate fixed effects of teachers encouraging students to believe in their ability to develop academic self-efficacy.
Список литературы
Adamuti-Trache, M. (2013), Language ac-quisition among adult immigrants in Canada: The effect of premigration language capital. Adult Edu-cation Quarterly, 63(2), 103-126. DOI: 10.1177/0741713612442804.
Adamuti-Trache, M. and Sweet, R. (2010), Adult immigrants’ participation in Canadian edu-cation and training. Canadian Journal for the Study of Adult Education, 22(2), 1-26.
Adamuti-Trache, M., Anisef, P. and Sweet, R. (2018), Differences in language proficiency and learning strategies among immigrant women to Canada. Journal of Language, Identity & Education, 17(1), 16-33. DOI: 10.1080/15348458.2017.1390433.
Bandura, A. (1986), Social foundations of thought and action. Prentice-Hall.
Boeren, E., Nicaise, I. and Baert, H. (2010), Theoretical models of participation in adult educa-tion: The need for an integrated model. Interna-tional Journal of Lifelong Education, 29(1), 45-61. DOI: 10.1080/02601370903471270.
Cebolla-Boado, H. and Soysal, Y.N. (2018), Educational optimism in China: Migrant selectivi-ty or migration experience? Journal of Ethnic and Migration Studies, 44(13), 2107-2126. DOI: 10.1080/1369183X.2017.1417825.
Chiswick, B.R. and Miller, P.W. (2015), In-ternational migration and the economics of lan-guage. In Handbook of the economics of interna-tional migration, 1, 211-269. North-Holland.
Chui, T.W. (2011), Immigrant women. Sta-tistics Canada.
Connell, J.P. and Wellborn, J.G. (1991), Competence, autonomy, and relatedness: A moti-vational analysis of self-system processes. In M. R. Gunnar & L. A. Sroufe (Eds.), The Minnesota sym-posia on child psychology, 23. 43-77. Lawrence Erlbaum Associates, Inc.
Council of Europe (2001), Common Europe-an framework of reference for languages: Learning, teaching, assessment. Cambridge University Press.
Cummins, J. (1981), Age on arrival and im-migrant second language learning in Canada: A reassessment. Applied Linguistics, 2(2), 132-149.
Epskamp, S. (2015), semPlot: Unified visual-izations of structural equation models. Structural Equation Modeling: A Multidisciplinary Journal, 22(3), 474-483. DOI:10.1080/10705511.2014.937847.
Fredericks, J.A., Blumenfeld, P.C. and Paris, A.H. (2004), School enthusiasm: Potential of the concept, state of the evidence. Review of Educa-tional Research, 74(1), 59-109.
Giddens, A. (1984), The constitution of socie-ty: Outline of the theory of structuration. University of California Press.
Goldin, C. (2016), Human capital. In C. Diebolt & M. Haupert (Eds.), Handbook of Clio-metrics, 55-86). Springer Verlag.
Isphording, I. (2015), “What drives the lan-guage proficiency of immigrants?” IZA World of Labor, 177, 1-10.
Manninen, J., and Meriläinen, M. (2011), Benefits of lifelong learning. BeLL Survey Results Retrieved from http://www.bell-project.eu/cms/wp-content/uploads/2014/06/BeLL-Survey-results.pdf.
Martin, A.J. and Dowson, M. (2009), Inter-personal relationships, motivation, engagement and achievement: Yields for theory, current issues and educational practice. Review of Educational Research, 79 (1), 327-365. https://doi.org/10.3102/0034654308325583.
Martinovic, B., Van Tubergen, F. and Maas, I. (2009), Changes in immigrants’ social integra-tion during the stay in the host country: The case of non-Western immigrants in the Netherlands. Social Science Research, 38(4), 870-882. https://doi.org/10.1016/j.ssresearch.2009.06.001.
McQuaid, R., Raeside, R., Canduela, J., Egdell, V., Lindsay, C. and Berry, C. (2012), En-gaging low skilled employees in workplace learning. UK Commission for Employment and Skills (UKCES), Evidence Report 43.
Naidoo, L. (2009), Developing social inclu-sion through after‐school homework tutoring: A study of African refugee students in Greater West-ern Sydney. British Journal of Sociology of Educa-tion, 30(3), 261-273. DOI: 10.1080/01425690902812547.
Nilsson, A. and Nyström, Ö. (2016), Flyktingkrisen och den svenska modellen [The Ref-ugee crises and the Swedish Welfare Model]. Ce-landers förlag.
Paradis, J., Soto-Corominas, A., Chen, X. and Gottardo, A. (2020), How language environ-ment, age, and cognitive capacity support the bi-lingual development of Syrian refugee children recently arrived in Canada. Applied Psycholinguis-tics, 1-27. doi.org/10.1017/S014271642000017X.
R Core Team (2018), R: A language and en-vironment for statistical computing.
R Foundation for Statistical Computing.
Revelle, W. (2018), Psych: Procedures for personality and psychological research. Northwest-ern University.
Rose, D. and Harrison, E. (2007), The Euro-pean socio-economic classification: A new social class schema for comparative European research. European Societies, 9(3), 459-490. https://doi.org/10.1080/14616690701336518.
Rosseel, Y. (2012), Lavaan: An R package for structural equation modeling and more. Version 0.5-12 (BETA). Journal of Statistical Software, 48(2), 1-36.
Sandwall, K. (2013), Att hantera praktiken: om sfi-studerandes möjligheter till interaktion och lärande på praktikplatser [Doctoral dissertation, Göteborgs Universitet]. Database.
Wedin, Å. and Norlund Shaswar, A. (2019), Whole class interaction in the adult L2-classroom: The case of Swedish for immigrants. Apples: Jour-nal of Applied Language Studies, 13(2), 17-34.
Wehlage, G.G., Rutter, R.A., Smith, G.A., Lesko, N.L. and Fernandez, R.R. (1989), Reducing the risk: Schools as communities of support. Farmer Press.
West, S. G., Taylor, A. B. and Wu, W. (2012), Model fit and model selection in structural equation modeling. Handbook of Structural Equa-tion Modeling, 1, 209-231.
Wrigley, H. (2013), New to literacy: Chal-lenges facing immigrants with minimal prior schooling. Perspectives on Language and Literacy, 39(2), 31–35.
Öbrink Hobzová, M. (2021), Development and current challenges of language courses for immigrants in Sweden. Journal of Adult and Con-tinuing Education, 27(1), 84-99.